Kaapana
Kaapana (from the hawaiian word kaʻāpana, meaning “distributor” or “part”) is an open source toolkit for state of the art platform provisioning in the field of medical data analysis. The applications comprise AI-based workflows and federated learning scenarios with a focus on radiological and radiotherapeutic imaging. Obtaining large amounts of medical data necessary for developing and training modern machine learning methods is an extremely challenging effort that often fails in a multi-center setting, e.g. due to technical, organizational and legal hurdles. A federated approach where the data remains under the authority of the individual institutions and is only processed on-site is, in contrast, a promising approach ideally suited to overcome these difficulties.
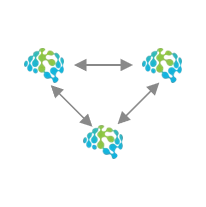
Federated Concept
The goal of Kaapana is to provide a framework and a set of tools for sharing data processing algorithms, for standardized workflow design and execution as well as for performing distributed method development. This will facilitate data analysis in a compliant way enabling researchers and clinicians to perform large-scale multi-center studies.

Open Source Technologies
By adhering to established standards and by adopting widely used open technologies for private cloud development and containerized data processing, Kaapana integrates seamlessly with the existing clinical IT infrastructure, such as the Picture Archiving and Communication System (PACS), and ensures modularity and easy extensibility.